The Role of Data Analytics in Transforming Strategic Financial Decision-Making
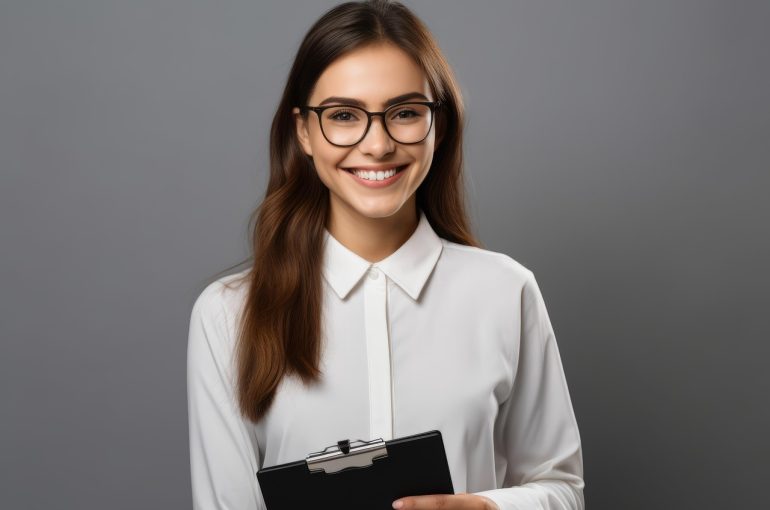
The Role of Data Analytics in Transforming Strategic Financial Decision-Making
Introduction to Data Analytics in Finance
Understanding Data Analytics
Data analytics refers to the process of examining datasets to draw conclusions about the information they contain. It involves the use of specialized systems and software to analyze data and is crucial in various industries, including finance. In finance, data analytics helps organizations make informed decisions by providing insights into financial trends, risks, and opportunities.
Evolution of Data Analytics in Finance
The evolution of data analytics in finance has been driven by technological advancements and the increasing availability of data. Initially, financial analysis relied heavily on manual processes and basic statistical methods. With the advent of computers and sophisticated software, financial institutions began to adopt more advanced analytical techniques. The rise of big data and machine learning has further transformed the landscape, enabling more precise and predictive financial analysis.
Key Technologies and Tools
Several key technologies and tools are integral to data analytics in finance. These include:
- Big Data Technologies: Tools like Hadoop and Spark allow for the processing and analysis of large volumes of data, which is essential for financial institutions dealing with vast amounts of information.
- Machine Learning Algorithms: These algorithms enable predictive analytics, helping financial analysts forecast market trends and assess risks.
- Data Visualization Tools: Software such as Tableau and Power BI help in visualizing complex data sets, making it easier for decision-makers to understand and interpret financial data.
- Cloud Computing: Cloud platforms provide scalable and flexible resources for storing and processing financial data, facilitating real-time analytics.
Applications in Financial Decision-Making
Data analytics is applied in various aspects of financial decision-making, including:
- Risk Management: By analyzing historical data and identifying patterns, financial institutions can better assess and manage risks.
- Fraud Detection: Advanced analytics can detect unusual patterns and anomalies in transactions, helping to prevent fraudulent activities.
- Investment Strategies: Data analytics provides insights into market trends and asset performance, aiding in the development of effective investment strategies.
- Customer Insights: Analyzing customer data helps financial institutions understand client behavior and preferences, leading to improved customer service and personalized offerings.
Challenges and Considerations
While data analytics offers significant benefits, there are challenges and considerations to keep in mind:
- Data Privacy and Security: Ensuring the privacy and security of financial data is paramount, given the sensitive nature of the information.
- Data Quality: The accuracy and reliability of data are crucial for effective analysis. Poor data quality can lead to incorrect conclusions and decisions.
- Integration with Legacy Systems: Many financial institutions face challenges in integrating new data analytics tools with existing legacy systems.
- Skill Gaps: There is a growing demand for skilled data analysts and data scientists in the finance sector, highlighting the need for training and development.
Historical Context: Evolution of Financial Decision-Making
Early Financial Decision-Making Practices
In the early stages of financial decision-making, practices were largely informal and based on rudimentary methods. Decisions were often made by individuals or small groups relying on personal judgment, intuition, and basic arithmetic. The lack of standardized financial systems meant that decisions were heavily influenced by personal relationships and subjective assessments. Record-keeping was minimal, often limited to simple ledgers, and financial analysis was not a formalized process.
The Advent of Double-Entry Bookkeeping
The introduction of double-entry bookkeeping in the 15th century marked a significant advancement in financial decision-making. This system, which originated in Italy, provided a more structured approach to recording financial transactions. It allowed for a clearer understanding of a business’s financial position and facilitated more informed decision-making. The double-entry system laid the groundwork for modern accounting practices, enabling businesses to track assets, liabilities, and equity systematically.
The Industrial Revolution and Financial Management
The Industrial Revolution in the 18th and 19th centuries brought about significant changes in financial decision-making. As businesses grew in size and complexity, there was a greater need for formal financial management. The rise of joint-stock companies and the expansion of capital markets necessitated more sophisticated financial analysis and planning. Financial managers began to emerge as key figures in organizations, tasked with optimizing resource allocation and ensuring financial stability.
The Emergence of Modern Financial Theories
The 20th century saw the development of modern financial theories that transformed decision-making processes. The introduction of concepts such as the time value of money, risk management, and portfolio theory provided a theoretical framework for financial analysis. These theories enabled decision-makers to evaluate investment opportunities more rigorously and to balance risk and return effectively. The development of financial models and quantitative techniques further enhanced the ability to make strategic financial decisions.
The Digital Revolution and Financial Decision-Making
The digital revolution of the late 20th and early 21st centuries has had a profound impact on financial decision-making. The advent of computers and the internet has facilitated the collection, storage, and analysis of vast amounts of financial data. This has led to the development of sophisticated financial software and analytics tools that provide real-time insights into financial performance. The ability to process large datasets quickly and accurately has transformed the way financial decisions are made, enabling more precise forecasting and strategic planning.
The Role of Data Analytics in Contemporary Financial Decision-Making
In recent years, data analytics has become an integral part of financial decision-making. Advanced analytics techniques, such as machine learning and artificial intelligence, are being used to uncover patterns and trends in financial data that were previously undetectable. These insights are helping organizations to make more informed and strategic financial decisions. Data analytics is also enabling more personalized financial services, as companies can tailor their offerings to meet the specific needs of individual clients. The integration of data analytics into financial decision-making processes is continuing to evolve, driving innovation and efficiency in the financial sector.
Key Components of Data Analytics in Finance
Data Collection and Integration
Data collection and integration form the foundation of data analytics in finance. This involves gathering data from various sources such as financial statements, market data, customer transactions, and external economic indicators. The integration process ensures that this data is consolidated into a unified system, allowing for seamless analysis. Effective data collection and integration require robust data management systems that can handle large volumes of data and ensure data quality and consistency.
Data Warehousing
Data warehousing is a critical component that involves storing and managing large sets of structured and unstructured data. Financial institutions use data warehouses to centralize data from different sources, making it accessible for analysis and reporting. A well-designed data warehouse supports complex queries and provides a historical record of financial data, which is essential for trend analysis and forecasting.
Data Mining and Pattern Recognition
Data mining involves exploring large datasets to identify patterns, correlations, and trends that can inform strategic financial decisions. Pattern recognition techniques help in detecting anomalies, predicting future trends, and uncovering hidden insights. In finance, data mining is used for credit scoring, fraud detection, and investment analysis, among other applications.
Predictive Analytics
Predictive analytics uses statistical algorithms and machine learning techniques to forecast future financial outcomes based on historical data. This component is crucial for risk management, investment strategy development, and financial planning. Predictive models can help financial institutions anticipate market movements, assess credit risk, and optimize asset allocation.
Real-Time Analytics
Real-time analytics enables financial institutions to process and analyze data as it is generated, providing immediate insights and facilitating quick decision-making. This is particularly important in high-frequency trading, fraud detection, and customer service optimization. Real-time analytics requires advanced computing infrastructure and efficient data processing techniques to handle the velocity and volume of incoming data.
Visualization and Reporting
Data visualization and reporting tools transform complex data sets into intuitive graphical representations, making it easier for decision-makers to understand insights and trends. Dashboards, charts, and graphs are commonly used to present financial data in a clear and concise manner. Effective visualization and reporting enhance communication and support strategic decision-making by providing stakeholders with actionable insights.
Machine Learning and Artificial Intelligence
Machine learning and artificial intelligence (AI) are increasingly integral to data analytics in finance. These technologies enable the automation of complex analytical tasks, improve the accuracy of predictive models, and enhance decision-making processes. AI-driven analytics can optimize trading strategies, personalize customer experiences, and streamline operations, providing a competitive edge in the financial industry.
Risk Management and Compliance
Data analytics plays a vital role in risk management and compliance by identifying potential risks and ensuring adherence to regulatory requirements. Advanced analytics can assess credit risk, market risk, and operational risk, helping financial institutions mitigate potential losses. Compliance analytics ensure that financial activities align with legal and regulatory standards, reducing the risk of penalties and reputational damage.
Impact of Data Analytics on Strategic Financial Decisions
Enhanced Decision-Making Accuracy
Data analytics significantly enhances the accuracy of strategic financial decisions by providing comprehensive insights derived from vast amounts of data. By leveraging advanced analytical tools, financial managers can identify patterns, trends, and anomalies that were previously undetectable. This leads to more informed decision-making processes, reducing the likelihood of errors and increasing the precision of financial forecasts and strategies.
Improved Risk Management
Data analytics plays a crucial role in improving risk management within financial decision-making. By analyzing historical data and current market trends, organizations can better predict potential risks and develop strategies to mitigate them. Predictive analytics, in particular, allows financial leaders to anticipate market fluctuations and adjust their strategies accordingly, ensuring that the organization is better prepared for potential financial challenges.
Cost Optimization
Through data analytics, companies can identify areas where costs can be reduced without compromising on quality or efficiency. By analyzing spending patterns and operational data, financial managers can pinpoint inefficiencies and implement cost-saving measures. This not only helps in optimizing the budget but also in reallocating resources to more strategic initiatives that drive growth and profitability.
Enhanced Financial Forecasting
Data analytics enhances financial forecasting by providing more accurate and reliable data-driven predictions. By utilizing historical data and real-time information, financial analysts can create more precise models that predict future financial performance. This allows organizations to plan more effectively, allocate resources efficiently, and set realistic financial goals that align with their strategic objectives.
Real-Time Financial Monitoring
The integration of data analytics into financial decision-making enables real-time monitoring of financial performance. This allows organizations to quickly identify and respond to any deviations from expected financial outcomes. Real-time insights facilitate agile decision-making, enabling companies to adapt their strategies promptly in response to changing market conditions or internal financial dynamics.
Strategic Resource Allocation
Data analytics aids in strategic resource allocation by providing insights into the most effective use of financial resources. By analyzing data on past investments and their outcomes, organizations can make informed decisions about where to allocate funds for maximum impact. This ensures that resources are directed towards initiatives that offer the highest potential for return on investment and align with the company’s long-term strategic goals.
Competitive Advantage
Organizations that effectively leverage data analytics in their financial decision-making processes gain a significant competitive advantage. By utilizing data-driven insights, companies can make more informed strategic decisions, anticipate market trends, and respond swiftly to changes in the business environment. This proactive approach enables organizations to stay ahead of competitors and capitalize on emerging opportunities in the market.
Case Studies: Successful Implementation of Data Analytics
Retail Industry: Walmart
Background
Walmart, one of the largest retail chains globally, has been at the forefront of leveraging data analytics to enhance its strategic financial decision-making. The company deals with massive volumes of data generated from its numerous stores and online platforms.
Implementation
Walmart implemented a sophisticated data analytics platform that integrates data from various sources, including sales transactions, customer feedback, and supply chain logistics. The platform uses advanced algorithms and machine learning models to analyze this data in real-time.
Outcomes
The implementation of data analytics has enabled Walmart to optimize its inventory management, reducing overstock and stockouts. It has also improved pricing strategies by analyzing competitor pricing and customer purchasing behavior. This has led to increased sales and improved profit margins.
Financial Services: JPMorgan Chase
Background
JPMorgan Chase, a leading global financial services firm, recognized the potential of data analytics in enhancing its financial decision-making processes. The firm deals with vast amounts of financial data, including transaction records, market trends, and customer information.
Implementation
The firm developed a comprehensive data analytics infrastructure that incorporates big data technologies and artificial intelligence. This infrastructure allows for the analysis of complex datasets to identify patterns and trends that inform strategic decisions.
Outcomes
Through data analytics, JPMorgan Chase has improved its risk management capabilities by predicting potential market risks and customer defaults. The firm has also enhanced its customer service by personalizing financial products and services, leading to increased customer satisfaction and retention.
Healthcare Sector: Kaiser Permanente
Background
Kaiser Permanente, a leading healthcare provider, sought to improve its financial decision-making by leveraging data analytics. The organization manages extensive patient data, including medical records, treatment outcomes, and billing information.
Implementation
Kaiser Permanente implemented a data analytics system that integrates clinical and financial data. The system uses predictive analytics to forecast patient demand and optimize resource allocation.
Outcomes
The use of data analytics has enabled Kaiser Permanente to reduce operational costs by streamlining processes and improving resource utilization. It has also enhanced patient care by identifying cost-effective treatment options and reducing unnecessary procedures.
Manufacturing Industry: General Electric (GE)
Background
General Electric, a multinational conglomerate, recognized the importance of data analytics in transforming its financial decision-making processes. The company operates in various sectors, including aviation, healthcare, and energy, generating vast amounts of operational data.
Implementation
GE developed a data analytics platform known as Predix, which collects and analyzes data from its industrial equipment. The platform uses advanced analytics to monitor equipment performance and predict maintenance needs.
Outcomes
The implementation of data analytics has allowed GE to reduce maintenance costs and improve equipment efficiency. By predicting equipment failures before they occur, GE has minimized downtime and enhanced productivity, leading to significant cost savings and increased profitability.
Challenges and Limitations in Data-Driven Financial Strategies
Data Quality and Integrity
Ensuring the quality and integrity of data is a fundamental challenge in data-driven financial strategies. Inaccurate, incomplete, or outdated data can lead to flawed analyses and misguided decisions. Financial data often comes from various sources, each with its own format and standards, making it difficult to maintain consistency and accuracy. Data cleansing and validation processes are essential but can be resource-intensive and time-consuming.
Data Privacy and Security
Data privacy and security are critical concerns in the financial sector, where sensitive information is frequently handled. The risk of data breaches and unauthorized access can undermine trust and lead to significant financial and reputational damage. Organizations must comply with stringent regulations, such as GDPR and CCPA, which impose strict requirements on data handling and protection. Balancing the need for comprehensive data analysis with privacy concerns is a persistent challenge.
Integration of Diverse Data Sources
Financial decision-making often requires the integration of diverse data sources, including structured data from financial statements and unstructured data from social media or news articles. The heterogeneity of these data sources poses significant integration challenges. Organizations must develop robust data integration frameworks to ensure seamless data flow and accessibility, which can be technically complex and costly.
Technological Infrastructure
Implementing data-driven strategies requires a robust technological infrastructure capable of handling large volumes of data and complex analytical processes. Many organizations face challenges in upgrading their existing systems or investing in new technologies due to budget constraints or legacy systems. The rapid pace of technological advancement also means that organizations must continuously adapt to stay competitive, which can be a daunting task.
Skill Gaps and Talent Shortage
The demand for skilled data analysts and financial experts who can interpret complex data sets and derive actionable insights is high. However, there is a notable skill gap in the industry, with many organizations struggling to find and retain qualified professionals. This talent shortage can hinder the effective implementation of data-driven strategies and limit an organization’s ability to leverage data analytics fully.
Overreliance on Quantitative Data
While quantitative data is crucial for financial decision-making, an overreliance on it can lead to a narrow focus that overlooks qualitative factors. Financial strategies that rely solely on quantitative data may miss important insights derived from qualitative analysis, such as market sentiment or consumer behavior. Balancing quantitative and qualitative data is essential for a comprehensive understanding of financial landscapes.
Resistance to Change
Organizational culture and resistance to change can be significant barriers to adopting data-driven financial strategies. Employees and management may be hesitant to shift from traditional decision-making processes to data-driven approaches due to a lack of understanding or fear of the unknown. Overcoming this resistance requires effective change management strategies, including education, training, and clear communication of the benefits of data analytics.
Cost Implications
The implementation of data-driven strategies can be costly, involving investments in technology, talent acquisition, and training. For some organizations, especially smaller ones, these costs can be prohibitive. Budget constraints may limit the ability to adopt advanced analytics tools or hire skilled professionals, impacting the overall effectiveness of data-driven financial strategies.
Future Trends in Data Analytics for Financial Decision-Making
Advanced Predictive Analytics
Predictive analytics is set to become more sophisticated, leveraging machine learning algorithms and artificial intelligence to forecast financial trends with greater accuracy. These advanced models will enable financial institutions to anticipate market shifts, customer behavior, and potential risks, allowing for more informed strategic decisions. The integration of real-time data feeds will further enhance the predictive capabilities, providing up-to-the-minute insights that can be crucial in volatile markets.
Real-Time Data Processing
The demand for real-time data processing is increasing as financial markets become more dynamic. Financial decision-makers will rely on systems that can process and analyze data instantaneously, allowing them to respond quickly to market changes. This trend is driven by the need for agility in decision-making, where delays can result in missed opportunities or increased risks. Technologies such as in-memory computing and edge analytics are expected to play a significant role in facilitating real-time data processing.
Integration of Alternative Data Sources
The use of alternative data sources, such as social media, satellite imagery, and IoT devices, is becoming more prevalent in financial analytics. These non-traditional data sources provide unique insights that can enhance the understanding of market conditions and consumer behavior. Financial institutions will increasingly integrate these data sources into their analytics frameworks to gain a competitive edge and uncover hidden opportunities.
Enhanced Data Visualization Tools
Data visualization tools are evolving to provide more intuitive and interactive ways to explore complex financial data. These tools will enable decision-makers to easily identify patterns, trends, and anomalies, facilitating a deeper understanding of the data. The use of augmented reality (AR) and virtual reality (VR) in data visualization is also expected to grow, offering immersive experiences that can enhance the analysis and presentation of financial information.
Increased Focus on Data Privacy and Security
As data analytics becomes more integral to financial decision-making, the focus on data privacy and security will intensify. Financial institutions will need to implement robust data governance frameworks to protect sensitive information and comply with regulatory requirements. The development of advanced encryption techniques and secure data sharing protocols will be critical in ensuring the integrity and confidentiality of financial data.
Democratization of Data Analytics
The democratization of data analytics refers to making advanced analytics tools accessible to a broader range of users within an organization. This trend will empower non-technical staff to leverage data insights in their decision-making processes, fostering a data-driven culture. User-friendly analytics platforms with self-service capabilities will be key in enabling this shift, allowing users to perform complex analyses without requiring extensive technical expertise.
Adoption of Cloud-Based Analytics Solutions
Cloud-based analytics solutions are gaining traction due to their scalability, flexibility, and cost-effectiveness. Financial institutions are increasingly adopting cloud platforms to store and analyze large volumes of data, enabling them to scale their analytics capabilities as needed. The cloud also facilitates collaboration and data sharing across different departments and locations, enhancing the overall efficiency of financial decision-making processes.
Conclusion: The Path Forward for Finance Professionals
Embracing Technological Advancements
Finance professionals must actively embrace technological advancements to remain competitive. The integration of artificial intelligence, machine learning, and advanced analytics tools is no longer optional but essential. These technologies enable the processing of vast amounts of data, providing insights that were previously unattainable. By leveraging these tools, finance professionals can enhance their decision-making processes, identify trends, and predict future financial scenarios with greater accuracy.
Continuous Learning and Skill Development
The rapid evolution of data analytics necessitates continuous learning and skill development. Finance professionals should seek to expand their knowledge in data science, programming, and statistical analysis. Engaging in professional development courses, attending workshops, and obtaining relevant certifications can help finance professionals stay abreast of the latest trends and tools in data analytics. This commitment to lifelong learning will ensure they remain valuable assets to their organizations.
Collaboration with Data Scientists
Collaboration between finance professionals and data scientists is crucial for maximizing the potential of data analytics. By working together, these professionals can combine their expertise to develop more sophisticated models and analyses. Finance professionals bring a deep understanding of financial principles and business acumen, while data scientists contribute technical skills and analytical prowess. This synergy can lead to more informed strategic financial decisions.
Ethical Considerations and Data Governance
As data analytics becomes more integral to financial decision-making, ethical considerations and data governance must be prioritized. Finance professionals should advocate for transparent data practices and ensure compliance with relevant regulations. Establishing robust data governance frameworks will help protect sensitive information and maintain the integrity of financial analyses. By upholding ethical standards, finance professionals can build trust with stakeholders and foster a culture of accountability.
Adapting to Changing Market Dynamics
The financial landscape is constantly evolving, and finance professionals must be agile in adapting to these changes. Data analytics provides the tools needed to navigate shifting market dynamics and respond proactively to emerging trends. By leveraging real-time data and predictive analytics, finance professionals can make strategic decisions that align with their organization’s goals and mitigate potential risks. This adaptability is key to maintaining a competitive edge in the ever-changing financial environment.
Related posts:
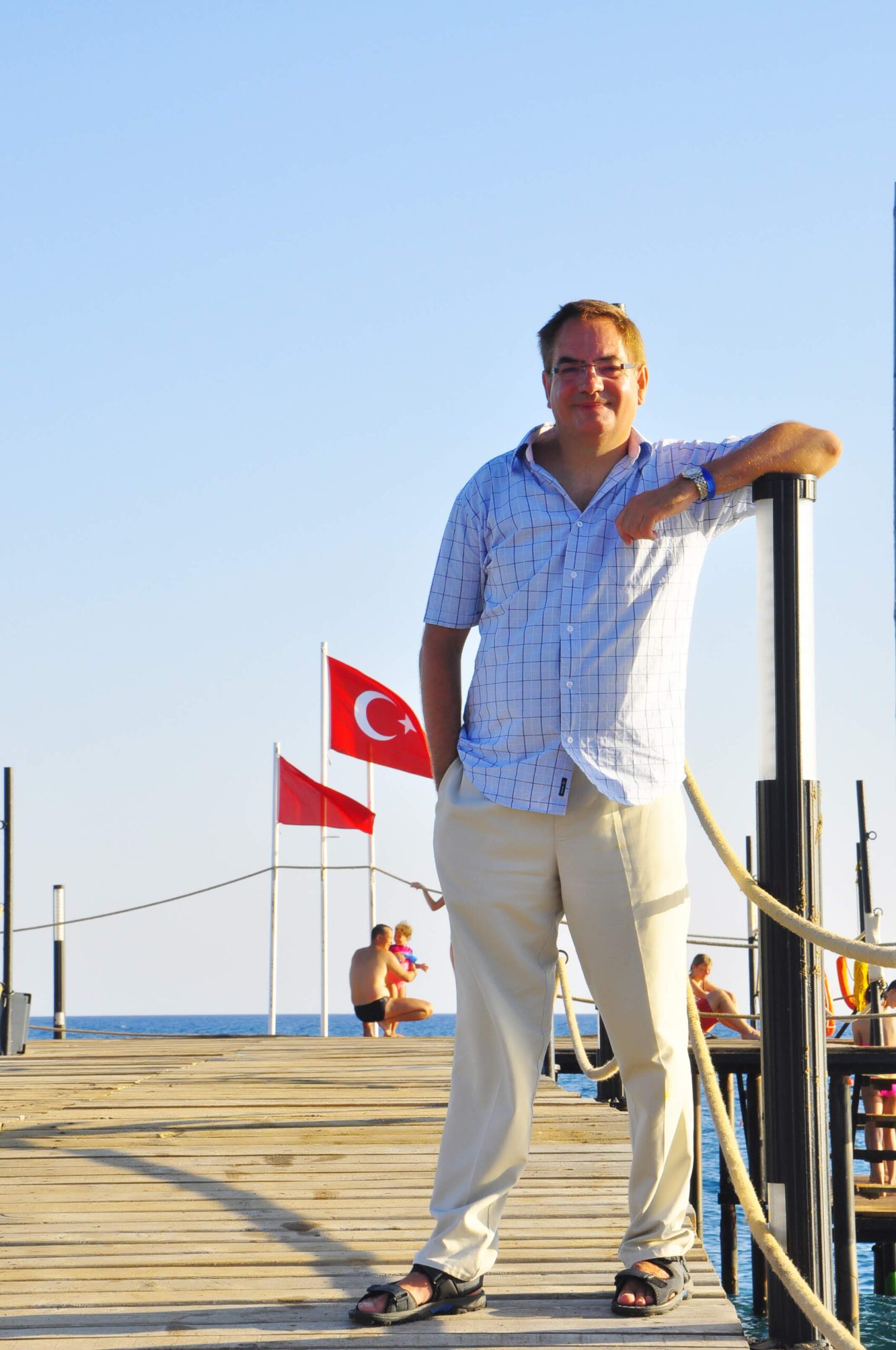
Adrian Lawrence FCA with over 25 years of experience as a finance leader and a Chartered Accountant, BSc graduate from Queen Mary College, University of London.
I help my clients achieve their growth and success goals by delivering value and results in areas such as Financial Modelling, Finance Raising, M&A, Due Diligence, cash flow management, and reporting. I am passionate about supporting SMEs and entrepreneurs with reliable and professional Chief Financial Officer or Finance Director services.