Risk Assessment Strategies for Financial Controllers
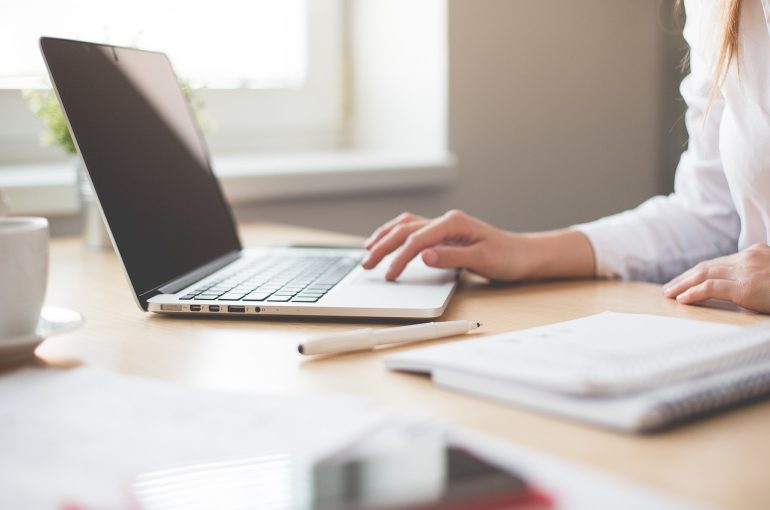
Risk Assessment Strategies for Financial Controllers
Introduction to Risk Assessment in the Digital Age
The Evolution of Risk Assessment
Risk assessment has undergone significant transformation with the advent of digital technologies. Traditionally, risk assessment in financial contexts relied heavily on manual processes, historical data analysis, and expert judgment. However, the digital age has introduced new dimensions to risk assessment, enabling more dynamic, data-driven, and predictive approaches. This evolution is characterized by the integration of advanced technologies such as artificial intelligence, machine learning, and big data analytics, which have revolutionized the way financial controllers identify, evaluate, and manage risks.
The Impact of Digital Transformation on Risk Landscapes
Digital transformation has reshaped the risk landscapes that financial controllers must navigate. The proliferation of digital platforms, cloud computing, and mobile technologies has introduced new types of risks, including cybersecurity threats, data privacy concerns, and operational vulnerabilities. These digital risks are often more complex and interconnected than traditional financial risks, requiring a more comprehensive and agile approach to risk assessment. Financial controllers must now consider a broader spectrum of risks that extend beyond financial metrics to include technological, operational, and reputational factors.
Key Technologies Influencing Risk Assessment
Several key technologies are influencing the way risk assessment is conducted in the digital age:
Big Data Analytics
Big data analytics allows financial controllers to process and analyze vast amounts of data from diverse sources in real-time. This capability enhances the accuracy and timeliness of risk assessments, enabling more informed decision-making. By leveraging big data, financial controllers can identify patterns, trends, and anomalies that may indicate potential risks, allowing for proactive risk management.
Artificial Intelligence and Machine Learning
Artificial intelligence (AI) and machine learning (ML) are transforming risk assessment by providing predictive insights and automating complex analytical tasks. These technologies can identify emerging risks and predict their potential impact with greater precision. AI and ML algorithms can also continuously learn and adapt to new data, improving the effectiveness of risk assessment over time.
Blockchain Technology
Blockchain technology offers enhanced transparency and security in financial transactions, reducing the risk of fraud and errors. By providing a decentralized and immutable ledger, blockchain can improve the integrity of financial data and streamline risk assessment processes. Financial controllers can leverage blockchain to verify transactions and ensure compliance with regulatory requirements.
Challenges and Opportunities in Digital Risk Assessment
The digital age presents both challenges and opportunities for risk assessment. One of the primary challenges is the rapid pace of technological change, which can outstrip the ability of financial controllers to keep up with new risks and regulatory requirements. Additionally, the increasing complexity of digital ecosystems can make it difficult to identify and assess all potential risks.
However, the digital age also offers opportunities for more effective risk management. The availability of advanced technologies and data analytics tools enables financial controllers to adopt a more proactive and strategic approach to risk assessment. By embracing digital innovations, financial controllers can enhance their ability to anticipate and mitigate risks, ultimately improving organizational resilience and performance.
The Evolving Role of Financial Controllers
Traditional Responsibilities
Financial Reporting and Compliance
Financial controllers have traditionally been responsible for ensuring accurate financial reporting and compliance with regulatory standards. This involves preparing financial statements, managing audits, and ensuring adherence to accounting principles and regulations.
Budgeting and Forecasting
Another core responsibility has been the development and management of budgets and forecasts. Financial controllers analyze financial data to project future financial performance, helping organizations allocate resources effectively.
Internal Controls and Risk Management
Financial controllers have played a crucial role in establishing and maintaining internal controls to safeguard assets and ensure the integrity of financial information. This includes identifying potential risks and implementing measures to mitigate them.
Impact of Digital Transformation
Automation and Technology Integration
The advent of digital tools and automation has transformed the role of financial controllers. They now oversee the integration of advanced technologies such as AI and machine learning into financial processes, which streamlines operations and enhances accuracy.
Data Analytics and Insights
With access to vast amounts of data, financial controllers are increasingly leveraging data analytics to provide strategic insights. This shift enables them to move beyond traditional number-crunching to offer data-driven recommendations that support business decision-making.
Cybersecurity and Data Privacy
As financial data becomes more digitized, financial controllers are tasked with ensuring cybersecurity and data privacy. They must work closely with IT departments to protect sensitive financial information from cyber threats and ensure compliance with data protection regulations.
Strategic Leadership
Business Partnering
Financial controllers are evolving into strategic business partners, collaborating with other departments to drive organizational growth. They provide financial insights that inform strategic planning and help align financial goals with business objectives.
Change Management
In a rapidly changing digital landscape, financial controllers are increasingly involved in change management initiatives. They play a key role in guiding organizations through financial transformations, ensuring that changes are implemented smoothly and effectively.
Talent Development
The evolving role also includes a focus on talent development within the finance team. Financial controllers are responsible for nurturing skills in data analytics, technology, and strategic thinking, preparing their teams for the demands of the digital age.
Emerging Technologies in Risk Assessment
Artificial Intelligence and Machine Learning
Artificial Intelligence (AI) and Machine Learning (ML) are revolutionizing risk assessment by enabling financial controllers to analyze vast amounts of data with unprecedented speed and accuracy. AI algorithms can identify patterns and anomalies that may indicate potential risks, allowing for proactive risk management. Machine learning models can be trained to predict future risks based on historical data, providing financial controllers with insights that were previously unattainable. These technologies also facilitate real-time risk monitoring, enabling organizations to respond swiftly to emerging threats.
Blockchain Technology
Blockchain technology offers a decentralized and secure way to record transactions, which can significantly enhance the transparency and traceability of financial data. For risk assessment, blockchain can be used to verify the authenticity of transactions and ensure data integrity. This reduces the risk of fraud and errors, as every transaction is recorded in an immutable ledger. Financial controllers can leverage blockchain to streamline auditing processes and improve compliance with regulatory requirements, thereby mitigating operational risks.
Big Data Analytics
Big data analytics allows financial controllers to process and analyze large volumes of structured and unstructured data from various sources. By harnessing big data, organizations can gain deeper insights into market trends, customer behavior, and potential risk factors. Advanced analytics tools can identify correlations and causations that may not be apparent through traditional analysis methods. This enables more informed decision-making and enhances the ability to anticipate and mitigate risks.
Robotic Process Automation (RPA)
Robotic Process Automation (RPA) involves the use of software robots to automate repetitive and rule-based tasks. In risk assessment, RPA can be employed to automate data collection, processing, and reporting, reducing the likelihood of human error and increasing efficiency. By automating routine tasks, financial controllers can focus on more strategic activities, such as analyzing risk data and developing risk mitigation strategies. RPA also ensures consistency and accuracy in risk assessment processes.
Internet of Things (IoT)
The Internet of Things (IoT) refers to the network of interconnected devices that collect and exchange data. In the context of risk assessment, IoT devices can provide real-time data on various operational parameters, such as equipment performance, environmental conditions, and supply chain activities. This data can be used to identify potential risks and vulnerabilities, allowing organizations to take preventive measures. IoT also enables predictive maintenance, reducing the risk of equipment failure and associated financial losses.
Cloud Computing
Cloud computing offers scalable and flexible solutions for data storage and processing, which are essential for effective risk assessment. By leveraging cloud-based platforms, financial controllers can access and analyze data from anywhere, facilitating collaboration and decision-making. Cloud computing also supports the integration of various risk assessment tools and technologies, enabling a comprehensive approach to risk management. The scalability of cloud solutions ensures that organizations can adapt to changing risk landscapes and data requirements.
Data Analytics and Predictive Modeling
The Role of Data Analytics in Risk Assessment
Data analytics has become a cornerstone in the financial sector, offering financial controllers the ability to process vast amounts of data to identify potential risks. By leveraging data analytics, financial controllers can gain insights into patterns and trends that may not be immediately apparent through traditional methods. This capability allows for a more comprehensive understanding of the financial landscape, enabling proactive risk management.
Types of Data Analytics Used in Financial Risk Assessment
Descriptive Analytics
Descriptive analytics involves summarizing historical data to understand what has happened in the past. This type of analysis is crucial for financial controllers as it provides a baseline for understanding past performance and identifying trends that could indicate potential risks.
Diagnostic Analytics
Diagnostic analytics goes a step further by examining the reasons behind past outcomes. By identifying the causes of past financial discrepancies or anomalies, financial controllers can better understand the underlying factors contributing to risk, allowing for more informed decision-making.
Predictive Analytics
Predictive analytics uses statistical models and machine learning algorithms to forecast future outcomes based on historical data. This approach is particularly valuable for financial controllers as it enables them to anticipate potential risks and take preemptive measures to mitigate them.
Prescriptive Analytics
Prescriptive analytics provides recommendations for actions to take based on predictive insights. For financial controllers, this means not only identifying potential risks but also receiving guidance on the best course of action to address these risks effectively.
Predictive Modeling Techniques
Predictive modeling is a critical component of modern risk assessment strategies. It involves using various statistical techniques to predict future events or behaviors. Some of the most common predictive modeling techniques used in financial risk assessment include:
Regression Analysis
Regression analysis is used to identify the relationships between variables and predict future trends. In the context of financial risk assessment, regression models can help predict financial outcomes based on various risk factors.
Decision Trees
Decision trees are a popular tool for classification and regression tasks. They help financial controllers visualize decision-making processes and understand the potential impact of different risk factors on financial outcomes.
Neural Networks
Neural networks, inspired by the human brain, are used to model complex patterns and relationships in data. They are particularly useful for identifying non-linear relationships and can be applied to various risk assessment scenarios in finance.
Time Series Analysis
Time series analysis involves analyzing data points collected or recorded at specific time intervals. This technique is essential for financial controllers to forecast future financial performance and identify potential risks based on historical trends.
Benefits of Integrating Data Analytics and Predictive Modeling
Integrating data analytics and predictive modeling into risk assessment strategies offers numerous benefits for financial controllers. These include:
- Enhanced Accuracy: By leveraging advanced analytics and modeling techniques, financial controllers can achieve more accurate risk assessments, leading to better decision-making.
- Proactive Risk Management: Predictive modeling allows for the anticipation of potential risks, enabling financial controllers to implement measures to mitigate these risks before they materialize.
- Improved Efficiency: Automation of data analysis and modeling processes reduces the time and effort required for risk assessment, allowing financial controllers to focus on strategic decision-making.
- Data-Driven Insights: The integration of data analytics provides financial controllers with actionable insights, helping them to understand complex financial environments and make informed decisions.
Cybersecurity and Digital Risk Management
Understanding Cybersecurity Threats
Types of Cyber Threats
- Malware and Ransomware: Discuss the prevalence of malicious software and ransomware attacks, which can encrypt or steal sensitive financial data.
- Phishing Attacks: Explain how phishing schemes target financial controllers to gain unauthorized access to financial systems.
- Insider Threats: Highlight the risks posed by employees or contractors who may misuse their access to financial data.
Emerging Threats in the Digital Age
- AI-Powered Attacks: Explore how cybercriminals are using artificial intelligence to enhance the sophistication of their attacks.
- IoT Vulnerabilities: Discuss the risks associated with the increasing use of Internet of Things devices in financial operations.
Digital Risk Management Strategies
Implementing Robust Security Protocols
- Multi-Factor Authentication (MFA): Emphasize the importance of using MFA to secure access to financial systems.
- Encryption: Discuss the role of encryption in protecting sensitive financial data both in transit and at rest.
Regular Security Audits and Assessments
- Vulnerability Assessments: Explain the need for regular assessments to identify and mitigate potential security weaknesses.
- Penetration Testing: Describe how simulated cyberattacks can help test the effectiveness of security measures.
Employee Training and Awareness
- Cybersecurity Training Programs: Highlight the importance of regular training sessions to keep employees informed about the latest cyber threats.
- Creating a Security-Conscious Culture: Discuss strategies for fostering a culture where employees are vigilant and proactive about cybersecurity.
Leveraging Technology for Risk Management
Advanced Analytics and AI
- Predictive Analytics: Explain how predictive analytics can be used to identify potential risks before they materialize.
- AI-Driven Security Solutions: Discuss the use of AI in monitoring and responding to cybersecurity threats in real-time.
Cloud Security Solutions
- Secure Cloud Infrastructure: Highlight the benefits of using secure cloud services to protect financial data.
- Data Backup and Recovery: Discuss the importance of having robust data backup and recovery plans in place to mitigate the impact of cyber incidents.
Regulatory Compliance and Governance
Understanding Regulatory Requirements
- GDPR and Data Protection: Explain the implications of data protection regulations like GDPR on financial data management.
- Industry-Specific Regulations: Discuss the need for compliance with industry-specific regulations that govern financial data security.
Governance Frameworks
- Risk Management Frameworks: Highlight the role of frameworks like NIST and ISO in guiding cybersecurity and risk management efforts.
- Board-Level Oversight: Discuss the importance of having board-level oversight to ensure that cybersecurity and digital risk management are prioritized at the highest levels of the organization.
Integrating Artificial Intelligence in Risk Assessment
Understanding AI in Financial Risk Assessment
AI technologies, such as machine learning and natural language processing, are transforming the landscape of financial risk assessment. These technologies enable financial controllers to analyze vast amounts of data quickly and accurately, identifying patterns and anomalies that may indicate potential risks. AI systems can process both structured and unstructured data, providing a comprehensive view of financial activities and potential threats.
Benefits of AI in Risk Assessment
Enhanced Accuracy and Efficiency
AI-driven risk assessment tools can significantly enhance the accuracy and efficiency of risk evaluations. By automating data analysis, AI reduces the likelihood of human error and allows financial controllers to focus on strategic decision-making. AI algorithms can continuously learn and adapt, improving their predictive capabilities over time.
Real-Time Monitoring and Alerts
AI systems can provide real-time monitoring of financial transactions and activities, offering immediate alerts when suspicious behavior is detected. This capability allows financial controllers to respond swiftly to potential risks, minimizing the impact on the organization.
Predictive Analytics
AI’s predictive analytics capabilities enable financial controllers to anticipate future risks based on historical data and trends. By identifying potential threats before they materialize, organizations can implement proactive measures to mitigate risks.
Implementing AI in Risk Assessment
Data Integration and Management
Successful AI implementation requires the integration of diverse data sources. Financial controllers must ensure that data is accurate, complete, and accessible. Effective data management practices are essential to maximize the benefits of AI technologies.
Choosing the Right AI Tools
Selecting the appropriate AI tools is crucial for effective risk assessment. Financial controllers should evaluate AI solutions based on their specific needs, considering factors such as scalability, ease of integration, and the ability to handle complex data sets.
Training and Development
To fully leverage AI technologies, financial controllers and their teams need adequate training. Understanding how AI systems work and how to interpret their outputs is essential for making informed decisions. Continuous learning and development programs can help teams stay updated with the latest AI advancements.
Challenges and Considerations
Data Privacy and Security
Integrating AI in risk assessment raises concerns about data privacy and security. Financial controllers must ensure that AI systems comply with relevant regulations and protect sensitive information from unauthorized access.
Ethical Considerations
The use of AI in risk assessment also involves ethical considerations. Financial controllers should be aware of potential biases in AI algorithms and strive to ensure that AI-driven decisions are fair and transparent.
Balancing Human and AI Input
While AI offers significant advantages, human judgment remains crucial in risk assessment. Financial controllers should balance AI insights with their expertise, ensuring that AI complements rather than replaces human decision-making.
Case Studies: Successful Implementation of Innovative Strategies
Leveraging Artificial Intelligence for Predictive Risk Analysis
Background
A leading multinational bank sought to enhance its risk assessment processes by integrating artificial intelligence (AI) into its financial operations. The bank faced challenges in predicting market volatility and credit risks due to the sheer volume of data and the complexity of global financial markets.
Implementation
The bank implemented an AI-driven predictive analytics platform that utilized machine learning algorithms to analyze historical data and identify patterns indicative of potential risks. The platform was integrated with the bank’s existing risk management systems, allowing for real-time data processing and analysis.
Outcomes
The AI system significantly improved the bank’s ability to predict market fluctuations and credit risks, reducing the time required for risk assessments by 40%. The bank reported a 25% decrease in non-performing loans and enhanced its decision-making capabilities, leading to more informed investment strategies.
Blockchain for Enhanced Transparency and Security
Background
A financial services firm specializing in cross-border transactions faced issues with transparency and security, leading to increased operational risks and compliance challenges. The firm aimed to leverage blockchain technology to address these concerns.
Implementation
The firm adopted a blockchain-based platform to record and verify all transactions. This decentralized ledger ensured that all parties involved in a transaction had access to the same information, reducing the risk of discrepancies and fraud.
Outcomes
The implementation of blockchain technology resulted in a 30% reduction in transaction processing times and a 50% decrease in compliance-related incidents. The firm also experienced improved client trust and satisfaction due to the enhanced transparency and security of its operations.
Cloud-Based Risk Management Solutions
Background
A mid-sized insurance company struggled with outdated risk management systems that were costly to maintain and lacked scalability. The company decided to transition to a cloud-based risk management solution to improve efficiency and reduce costs.
Implementation
The company partnered with a cloud service provider to migrate its risk management operations to a cloud-based platform. This transition included the integration of advanced analytics tools and automated reporting features.
Outcomes
The cloud-based solution enabled the company to reduce its IT infrastructure costs by 35% and improve the scalability of its risk management operations. The company also benefited from enhanced data analytics capabilities, leading to more accurate risk assessments and improved policy pricing strategies.
Data Analytics for Proactive Risk Mitigation
Background
A global investment firm needed to enhance its risk mitigation strategies to better anticipate and respond to market changes. The firm decided to implement advanced data analytics to gain deeper insights into potential risks.
Implementation
The firm deployed a comprehensive data analytics platform that aggregated data from various sources, including market trends, economic indicators, and client portfolios. The platform utilized advanced algorithms to identify emerging risks and provide actionable insights.
Outcomes
The data analytics initiative enabled the firm to proactively address potential risks, resulting in a 20% reduction in portfolio volatility. The firm also improved its client advisory services by providing more tailored risk management solutions, enhancing client satisfaction and retention.
Future Trends and Challenges in Risk Assessment for Financial Controllers
Emerging Technologies and Their Impact
Artificial Intelligence and Machine Learning
The integration of artificial intelligence (AI) and machine learning (ML) into financial risk assessment is transforming how financial controllers identify and manage risks. AI and ML algorithms can analyze vast amounts of data at unprecedented speeds, uncovering patterns and anomalies that might be missed by human analysts. These technologies enable predictive analytics, allowing financial controllers to anticipate potential risks before they materialize. However, the challenge lies in ensuring the accuracy and reliability of AI-driven insights, as well as addressing ethical concerns related to data privacy and algorithmic bias.
Blockchain and Distributed Ledger Technology
Blockchain technology offers a new level of transparency and security in financial transactions, which can significantly enhance risk assessment processes. By providing an immutable record of transactions, blockchain can reduce the risk of fraud and errors. Financial controllers can leverage distributed ledger technology to streamline auditing processes and improve the accuracy of financial reporting. The challenge, however, is the integration of blockchain systems with existing financial infrastructures and the need for regulatory frameworks to govern their use.
Regulatory Changes and Compliance
Evolving Regulatory Landscape
The financial industry is subject to an ever-changing regulatory environment, with new rules and standards being introduced regularly. Financial controllers must stay abreast of these changes to ensure compliance and mitigate associated risks. This requires continuous monitoring of regulatory updates and the ability to adapt risk assessment strategies accordingly. The challenge is the complexity and volume of regulations, which can vary significantly across jurisdictions, making it difficult for financial controllers to maintain compliance on a global scale.
Data Privacy and Protection Regulations
With the increasing focus on data privacy, regulations such as the General Data Protection Regulation (GDPR) in Europe and the California Consumer Privacy Act (CCPA) in the United States have significant implications for risk assessment. Financial controllers must ensure that their risk management practices comply with these regulations, which involves safeguarding sensitive financial data and implementing robust data protection measures. The challenge is balancing the need for comprehensive data analysis with the requirement to protect individual privacy rights.
Cybersecurity Threats
Increasing Sophistication of Cyber Attacks
As financial systems become more digitized, they are increasingly vulnerable to cyber threats. Cybercriminals are employing more sophisticated techniques to breach financial systems, posing significant risks to financial controllers. Effective risk assessment strategies must include robust cybersecurity measures to protect against data breaches, ransomware attacks, and other cyber threats. The challenge is keeping pace with the rapidly evolving threat landscape and ensuring that cybersecurity defenses are continually updated and strengthened.
Integration of Cyber Risk into Financial Risk Assessment
Financial controllers must integrate cyber risk into their overall risk assessment frameworks. This involves understanding the potential financial impact of cyber incidents and developing strategies to mitigate these risks. The challenge is quantifying cyber risk in financial terms and ensuring that it is given appropriate consideration alongside traditional financial risks.
Data Analytics and Big Data
Leveraging Big Data for Risk Assessment
The explosion of big data presents both opportunities and challenges for financial controllers. By harnessing big data analytics, financial controllers can gain deeper insights into risk factors and improve decision-making processes. This involves analyzing diverse data sources, including market trends, customer behavior, and economic indicators. The challenge is managing the sheer volume and variety of data, ensuring data quality, and extracting meaningful insights that can inform risk assessment strategies.
Challenges in Data Integration and Management
Integrating and managing data from multiple sources is a significant challenge for financial controllers. Effective risk assessment requires a holistic view of financial data, which often involves consolidating information from disparate systems and platforms. The challenge is ensuring data consistency, accuracy, and accessibility while maintaining data security and compliance with regulatory requirements.
Related posts:
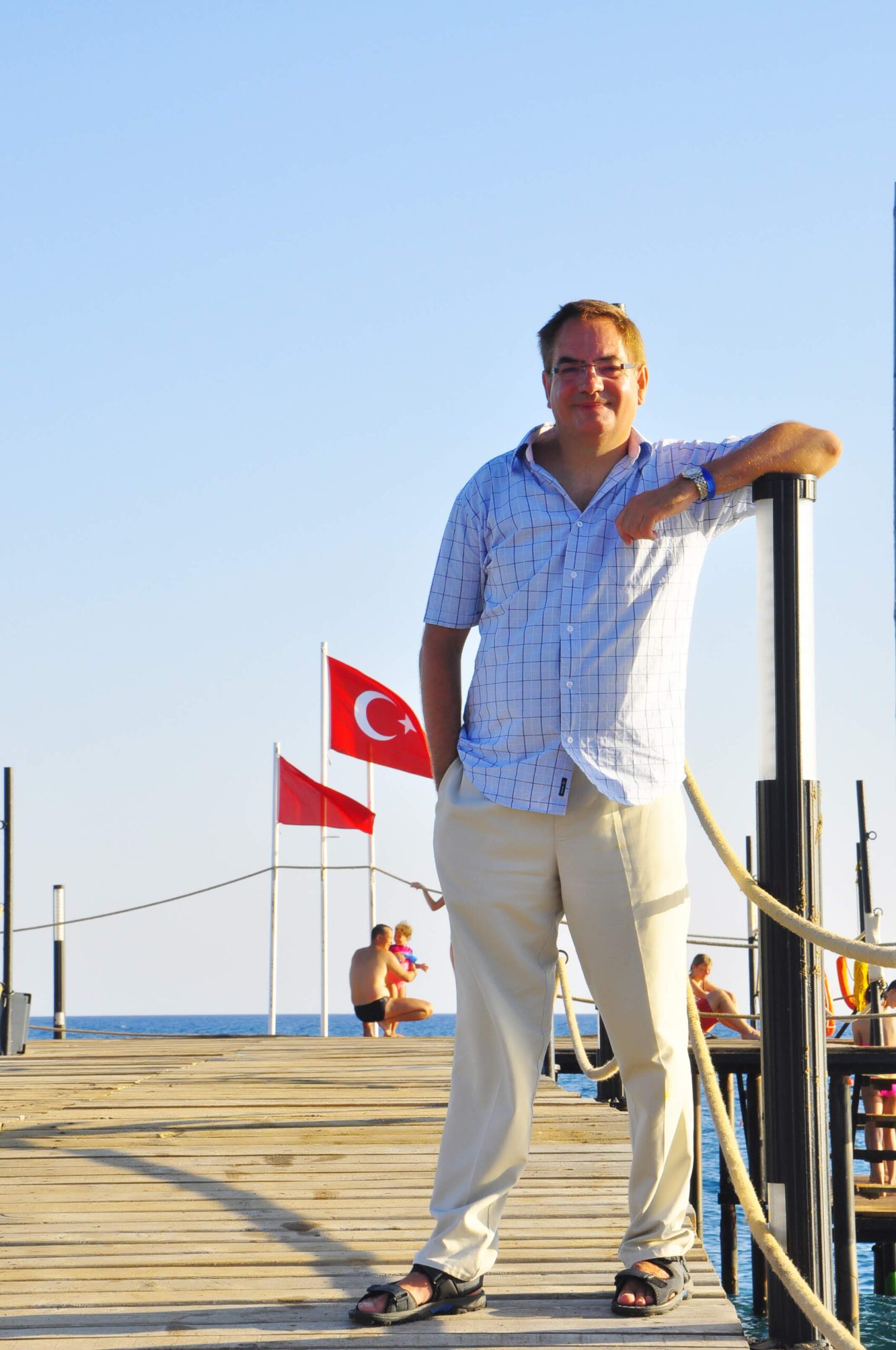
Adrian Lawrence FCA with over 25 years of experience as a finance leader and a Chartered Accountant, BSc graduate from Queen Mary College, University of London.
I help my clients achieve their growth and success goals by delivering value and results in areas such as Financial Modelling, Finance Raising, M&A, Due Diligence, cash flow management, and reporting. I am passionate about supporting SMEs and entrepreneurs with reliable and professional Chief Financial Officer or Finance Director services.